#49 - Speculative Swells and the Memecoin Aftermath
Research Study on Demand and Supply Shocks in Crypto
Stanford Blockchain Review
Volume 5, Article No. 9
📚Authors: Matt Stephenson — Pantera Research
Ally Zach — Pantera Research
🌟Technical Prerequisite: Intermediate
Introduction
For most people, unexpected disruptions in supply chains have little upside. However, for researchers, these disruptions offer valuable opportunities to understand market dynamics that are otherwise hard to disentangle. For instance, because prices and quantities are the observed outcomes of supply and demand, it's hard to know whether supply, demand, or both have an impact. This gives rise to the old saying "don't reason from a price change." But when one or the other factor suddenly changes in a predictable way, sometimes you can.
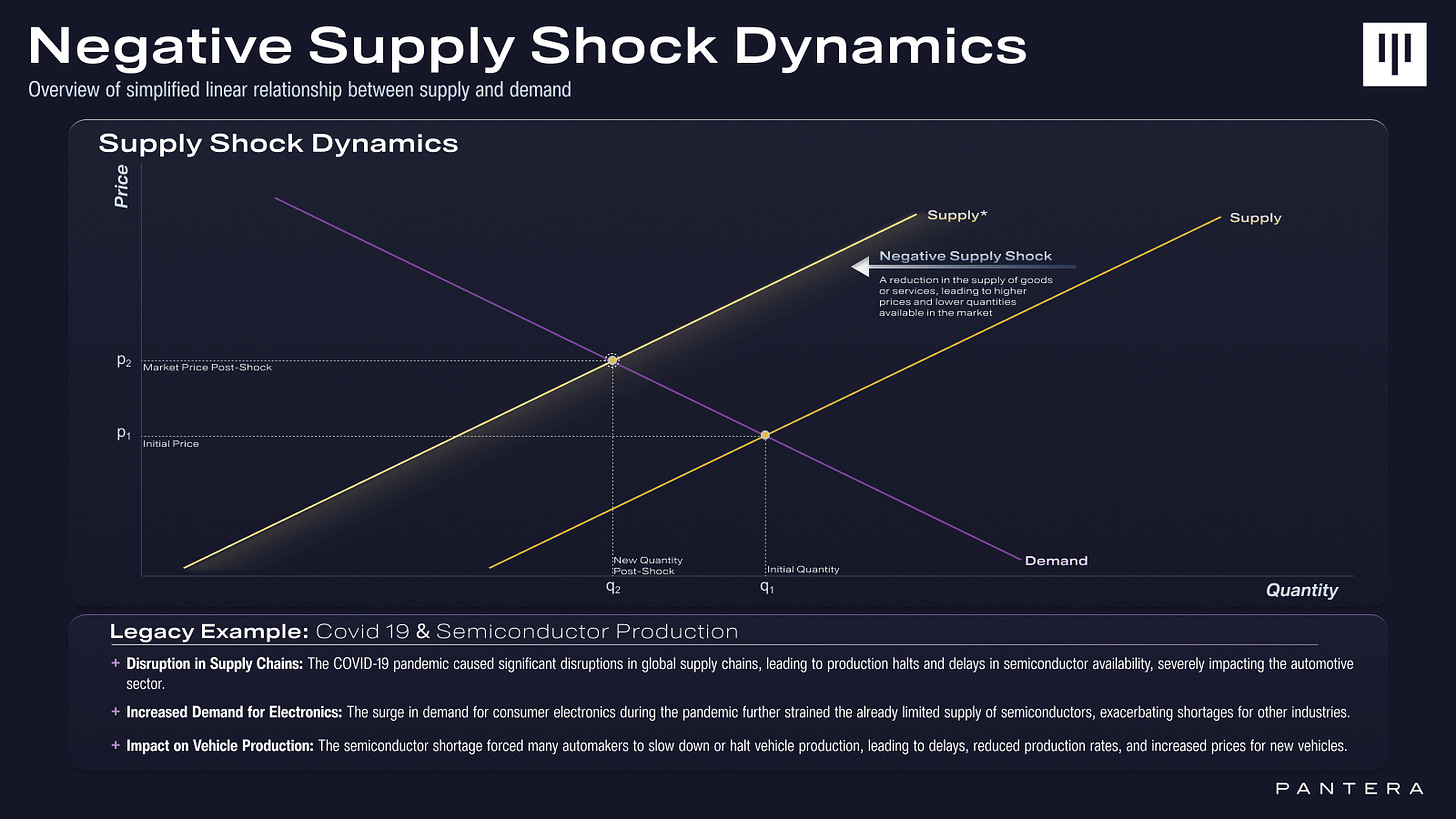
Blockchain networks operate with inherent capacity constraints similar to production lines. Each block has a fixed capacity for transaction data, rendering space a limited resource. As transaction demands escalate, the competition for block space intensifies, leading to potential network congestion.
In March, the implementation of EIP-4844 aimed to enhance network capacity and lower transaction costs for Ethereum’s Layer-2s, benefiting networks like Arbitrum and Optimism with significant gas price reductions [1]. However, shortly after this implementation, Base experienced a surge in gas prices, exceeding previous levels observed before EIP-4844 was introduced.
During this period, there was a notable increase in user activity on Base, primarily fueled by DeFi trading activities. This surge was particularly unexpected given that Base's ecosystem has been cultivated to favor consumer-oriented applications. Initially incubated by the Coinbase team, Base has benefitted from extensive marketing and branding efforts aimed at creating a chain that encourages creators, builders, and community engagement. As a result, the ecosystem predominantly consists of consumer apps, with the most successful applications, like Friend.tech, being consumer-oriented.
The reversal in user activity and sudden surge in trading on Base could potentially be attributed to a supply shock, induced by an unexpected external event that impacted the system’s supply chain. Such shocks can significantly alter availability and costs, fundamentally changing user behavior and network dynamics.
Catalyst Hunting
In order to qualify as a genuine supply shock, the event must be exogenous, unexpected, and sufficiently potent to disrupt established market dynamics.
Following the implementation of EIP-4844, one of the most significant changes was the sudden increase in DEX trading, which expanded beyond the typical stablecoins and ETH to encompass new tokens. Previously, trading on Base had predominantly focused on these categories, with meme tokens accounting for less than 15% on average of the weekly trading volume across all DEXs.
Historically, memecoin seasons are often ignited by a "beacon" token that captures significant market interest and sets new trading benchmarks. This phenomenon is likely driven by factors like information cascades [2]. On platforms like Crypto Twitter, successful trading stories are amplified, while failures are often ignored, leading to a skewed perception of potential gains. As traders observe and imitate the actions of others, assuming they have valuable insights, a self-reinforcing loop is created. This drives up the prices of memecoins rapidly and often leads to significant market volatility.
For example, in late 2023 on Solana, the dogwifhat token (WIF) rose from a market cap of less than $1 million to billions within months [3]. The success of WIF inspired a memecoin mania on Solana, marked by an increase in meme token launches and the development of memecoin infrastructure [4].
While memecoins have existed on Base since it first launched, none of them captured market mindshare until March of this year. Base's initial mainnet launch was fueled by a memecoin trading frenzy [5]. Prior to the official launch of the network, thousands of users migrated to Base to trade meme coins. The activity on these tokens tapered down as new applications started to launch. Inspired by a character from a popular book featuring Pepe, the BRETT token launched in late February to early March quickly made its mark on Base, achieving a market capitalization of $350 million well before significant meme trading activity took hold [5]. Its rapid rise not only distinguished it from typical market trends but also ignited a broader trading frenzy across the network.
The initial success of the BRETT token drew speculative traders through potential mimetic effects, attracting a new cohort of users more interested in meme trading than in engaging with the network's applications. Although this group's focus has remained narrow, it is valuable to explore the ripple effects of this meme frenzy on the existing user base of Base's ecosystem, particularly how their typical behaviors shifted in response to this event. That said, while surface-level data alone cannot confirm that the observed congestion directly resulted from the BRETT token event, it does encourage us to pursue a more detailed analysis to accurately assess the direct impacts on user behavior and demand.
The Experiment:
The primary objective of the proposed experiment is to analyze the supply and demand dynamics on Base, focusing on how gas fees (supply) and transaction activity (demand) interact before, during, and after the BRETT event. A critical aspect of this analysis involves isolating the impact of the BRETT launch from general market behavior.
To gain clear insights into market dynamics, we will exclude trading activity directly related to the BRETT token. Our analysis will concentrate on addresses that were active prior to the token’s launch in late February, enabling us to evaluate a consistent user base unaffected by the speculative interest that new tokens often generate. This approach ensures that our examination of broader user behavior on Base remains unbiased, not disproportionately influenced by those primarily interested in BRETT.
The Model:
In this study, we utilize a regression model with a core binary variable to analyze the impact of the BRETT launch. The selection of variables and their functions within the model are chosen to reflect the nuanced effects of this market event.
The model is defined as follows:
Where:
Average Gas Usage (𝑄𝑡): Represents the average gas usage at time 𝑡, serving as a key indicator of transaction complexity and network load.
Shock Indicator (𝐷𝑡): A binary variable that indicates whether the BRETT token event occurred (0 if before the launch, 1 otherwise).
Gas Fee (𝑃𝑡): Denotes the price of gas in gwei at time 𝑡.
Interaction Term (𝐷𝑡𝑃𝑡): Captures the interaction between the BRETT shock and the gas prices.
Transaction Count (𝑇𝑡): Denotes the number of transactions at time 𝑡, included to understand how variations in transaction volume influence network congestion and gas usage.
It's important to acknowledge that this model, in its current form, is somewhat simplistic and primarily serves to suggest shifts in demand related to this specific catalyst. The model does not account for potential endogeneity stemming from baseline conditions or other underlying trends, which could obscure the true causality and elasticity of demand prior to the event. For instance, omitted variables may exist, and there could be simultaneous causality between gas usage and fees, along with additional noise that could compromise the accuracy of our initial estimates.
However, this model enables us to determine whether the BRETT shock led to a statistically significant change in transaction behavior on Base, independent of BRETT's direct trading activities.
Regression Results
Looking at non-BRETT specific cohort of users from early January 2024 until the end of May on an hourly basis, we can deduce the following surrounding the launch and first run up of the BRETT token:
Following the Brett token's introduction on Base, there was a statistically significant behavioral shift among users in response to rising gas prices. The regression model indicated a significant negative interaction term (𝛽3=−0.333) which suggests that the increase in gas fees after the token's introduction likely deterred user transactions.
Specifically, the interaction term suggests that for a one standard deviation increase in gas prices (Δ𝑃=1.2×105 gwei) following the meme event, we can expect a decrease in gas usage, Δ𝑄, of 41.2k, which corresponds to 79% of the typical hourly standard deviation. In other words, according to the model, a one standard deviation increase in gas prices results in approximately a 0.79 standard deviation decrease in demand during a high congestion event.
Overall, the introduction of the memecoin beacon token, BRETT, had adverse ripple effects on Base's initial user base. The catalyst-induced congestion heightened this cohort's sensitivity to rising gas prices, making them more averse to transaction costs—even though these costs were close to levels observed prior to the implementation of EIP-4844.
Zooming Out
The ripple effects of BRETT on Base serves as an illustration of the broader vulnerabilities and adaptive behaviors characteristic of crypto ecosystems. This event underscores how emergent tokens, and more particularly unexpected events, can substantially affect transaction metrics, user behavior, and network stability, illustrating the rapid pace at which dynamics can evolve within a blockchain's operational framework.
This incident highlights the nuanced relationship between supply (in this case, network fees) and user demand, which is far from a simple linear equation. Demand can shift abruptly, as evidenced by the BRETT event, or evolve more gradually as the ecosystem matures. Such shifts underscore the complex interplay between network adjustments and user responses, which are not always predictable and can vary widely depending on external shocks or anticipated changes like network upgrades.
Looking ahead, as more exogenous events or known upgrades occur, understanding these underlying dynamics becomes vital. Recognizing the patterns and potential reactions of users to changes within the ecosystem can help in forecasting more genuine user dynamics and responses.
About The Authors
Matt Stephenson is Research Partner at Pantera. He has lectured at Columbia University on DAO governance, with a doctoral thesis focused on price discovery and endogenous value in NFT markets. He was previously Senior Mechanism Designer at BlockScience, working with organizations from Dapper Labs to MakerDAO. Matt has a PhD in Behavioral Economics & Strategy from Columbia University, Master’s in Strategy from Columbia Business School, and M.S. in Economics from the University of Warwick as a Fulbright Scholar. Follow him on X @stephensonhmatt
Ally Zach is a Research Engineer at Pantera Capital. Previously, she worked as an Enterprise Research Analyst at Messari, specializing in data-driven analytics. Ally holds an M.S. in Structural Engineering from the University of Illinois. Follow her on X @0xallyzach.
References
[1] https://eips.ethereum.org/EIPS/eip-4844
[2] https://www.jstor.org/stable/2951328
[3] https://x.com/dogwifcoin
[4] https://dune.com/ally/solana-memecoins
[5] https://www.coindesk.com/markets/2024/03/26/base-blockchain-transactions-jump-on-meme-coin-led-frenzy/